Speaker Details
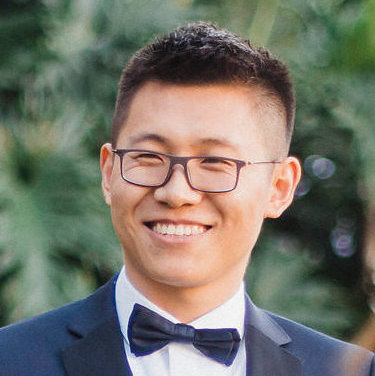
Xin (Eric) Wang
UC Santa Cruz
Xin (Eric) Wang is an Assistant Professor of Computer Science and Engineering at UC Santa Cruz and the Head of Research at Simular. His research interests include Natural Language Processing, Computer Vision, and Machine Learning, with an emphasis on Multimodal Reasoning and AI Agents. He worked at Google Research, Facebook AI Research (FAIR), Microsoft Research, and Adobe Research. Eric has served as Area Chair for conferences such as ACL, NAACL, EMNLP, ICLR, and NeurIPS, as well as a Senior Program Committee for AAAI and IJCAI. He organized workshops and tutorials at conferences such as ACL, NAACL, CVPR, and ICCV. He has received several awards and recognitions for his work, including CVPR Best Student Paper Award, Google Research Faculty Award, Amazon Alexa Prize Awards, Cisco Research Award, eBay Research Awards, and various faculty research awards from Adobe, Snap, Microsoft, Cybever, etc.
Talk
Title: What Is New in LLM Safety? A (Multimodal) Reasoning Perspective
Abstract: Recent advancements in Large Language Models (LLMs) have dramatically expanded their reasoning capabilities, enabling complex problem-solving and nuanced multimodal understanding. Yet, this growth introduces novel safety challenges, requiring deeper investigation into how reasoning influences safe and reliable interactions. This talk offers a unified perspective by discussing recent research that reveals interconnected aspects of model safety. We first introduce the concept of Multimodal Situational Safety, examining how situational awareness shapes a model’s ability to interpret contexts and respond safely in realistic settings. We then highlight the vulnerabilities in Large Reasoning Models (LRMs) such as R1, emphasizing their susceptibility to adversarial attacks and unique hidden risks. Lastly, we propose a novel benchmark for evaluating Multimodal Inconsistency Reasoning, critical for detecting contradictions and enhancing model reliability across modalities. By integrating these findings, we identify essential directions for the safer deployment of increasingly powerful LLMs and LRMs.